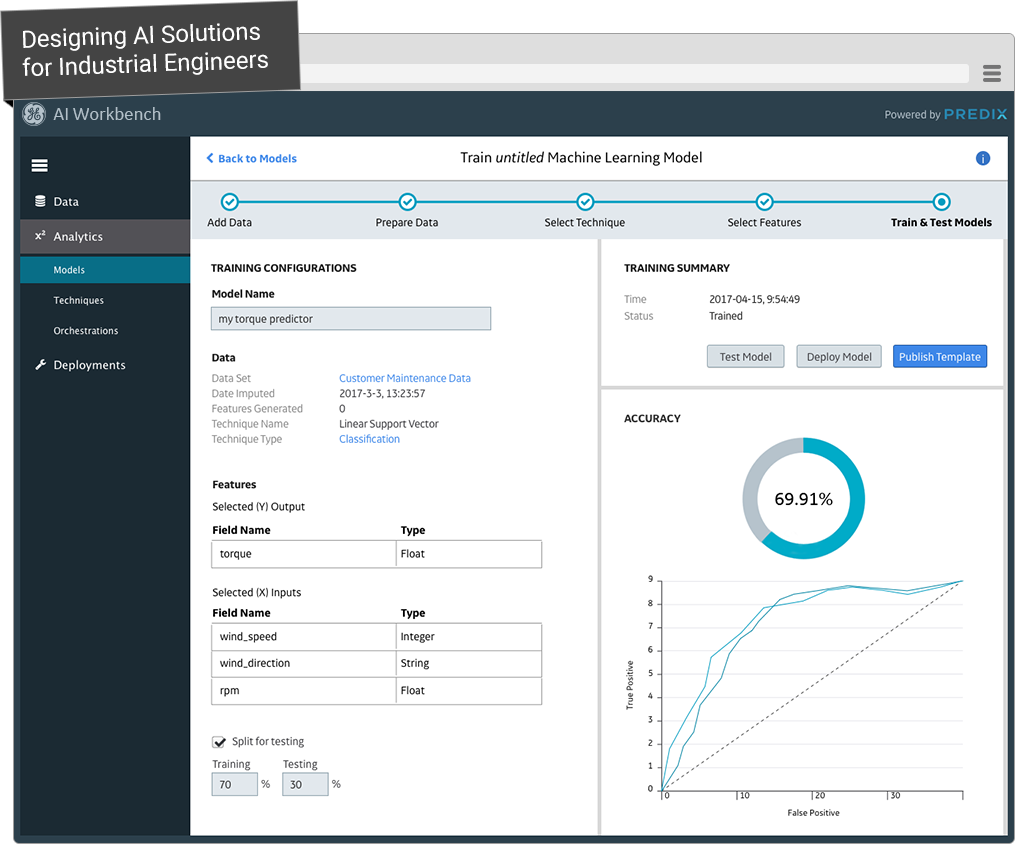
The goal is to get machine learning techniques into the hands of industrialists. The challenge is the complexity involved in accurately training machine learning algorithms. This typically requires data science expertise, but as more generalists become more tech savvy, the power of machine learning can be put into the hands of folks closer to the data. Operations Engineers are the users who are often the most familiar with data coming off the sensors of industrial equipment.
Product Strategy
As Senior Staff UX Interaction Designer at GE Digital, I worked closely with stakeholders (customers, product managers, and business leaders) to help define product roadmaps. I worked closely with international scrum teams (California, Bangalore, New York) to ensure consistent user experience, and ensured that the conceptual models and tools were intuitive to users and provided customer value.
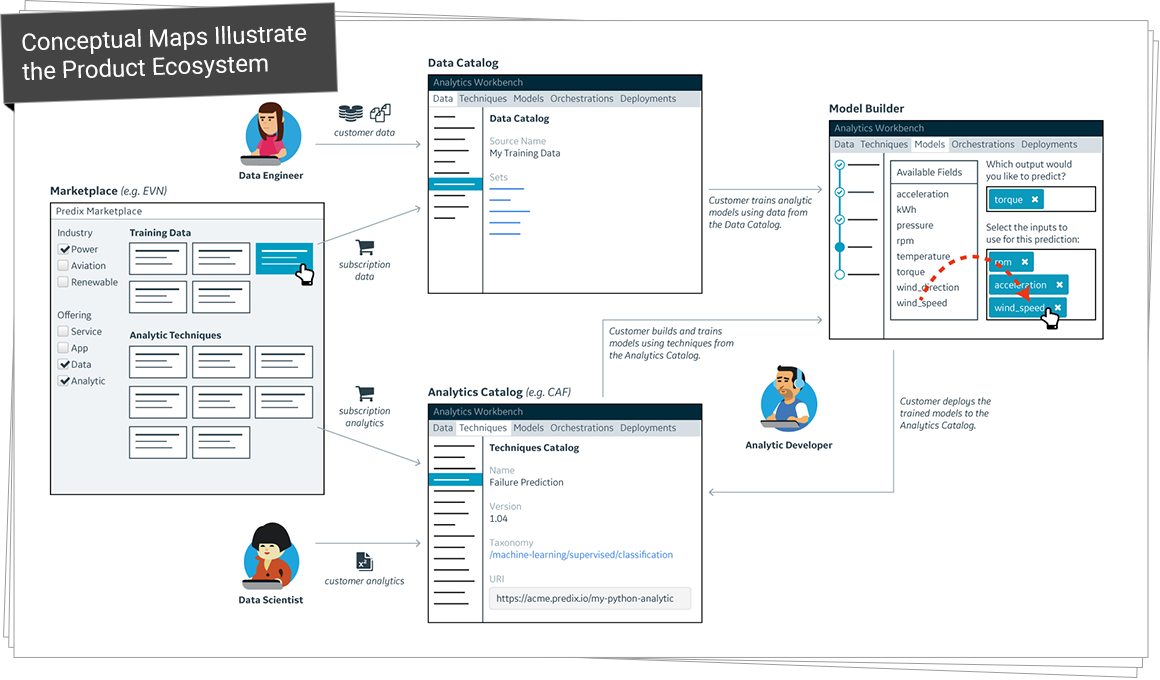
Storyboarding and concept flows help stakeholders, product managers, and engineering teams quickly grasp the high-level user flow of the product.
Contextual Observation
To better understand users, I worked closely with a UX Researcher to visit customer sites to observe user workflows and gain critical insights into user behaviors. Industry roles for this line of products include Reliability Engineers, Data Analysts, and Data Scientists.
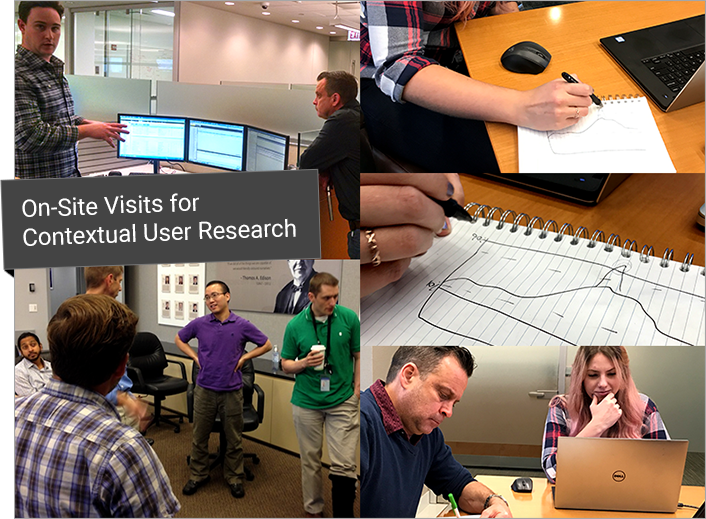
Data Curation
Interviews with users and subject matter experts revealed that data exploration and data curation is a critical part of the process. Data visualization tools let users evaluate data before and after model training.
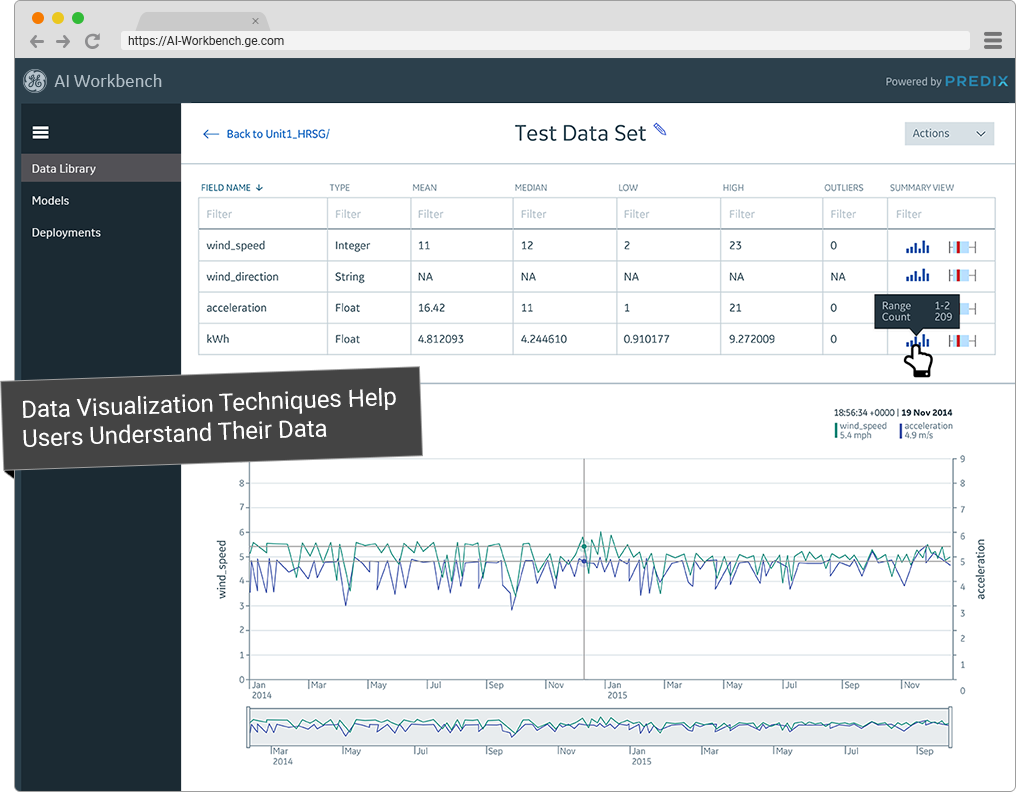
Equipment Dashboards
The output of the deployed AI models feed into prognostic dashboards, giving users insights to make better on-the-job decisions.
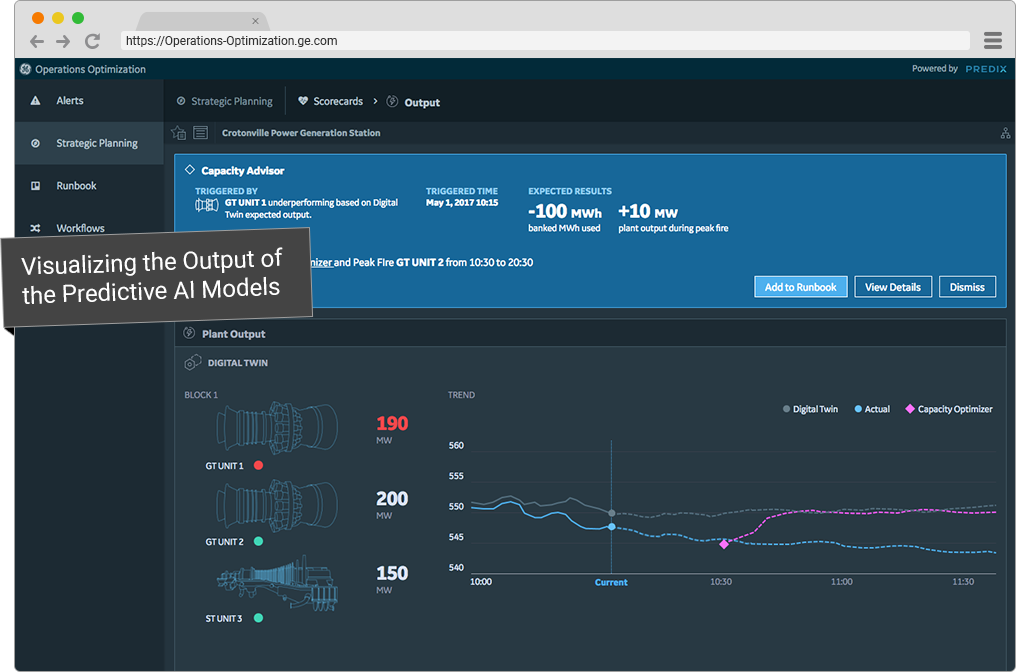
The primary personas for these dashboards are Reliability Engineers and Performance Analysts. The visual look for the equipment monitoring dashboards is dark because industrial equipment monitoring facilities are often in lowlight environments.
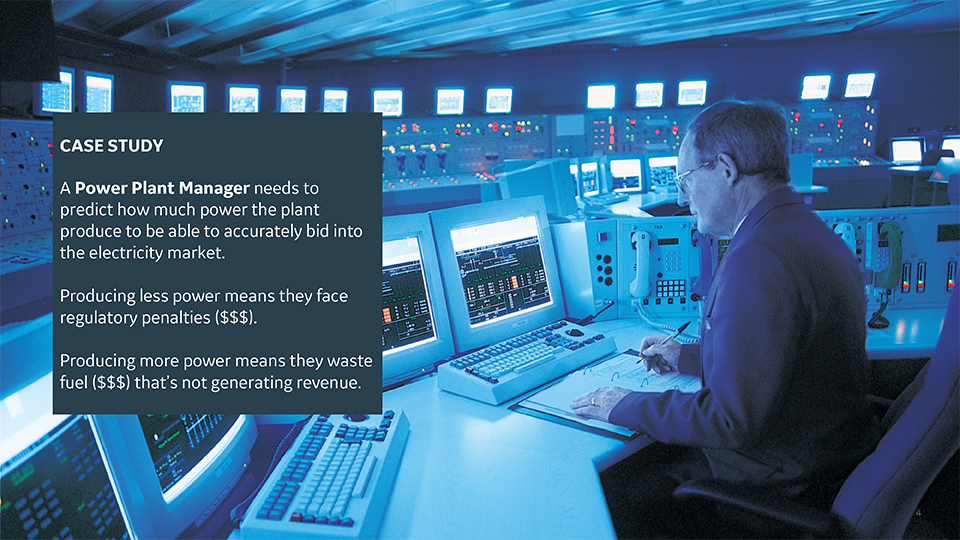
The data science process is very iterative, and often never complete because machine learning models might get “stale” due to "data drift". I led the team in designing monitoring tools so that Operators can ensure that the predicted results are close to actual results. If not, the machine learning model may have to be retrained.
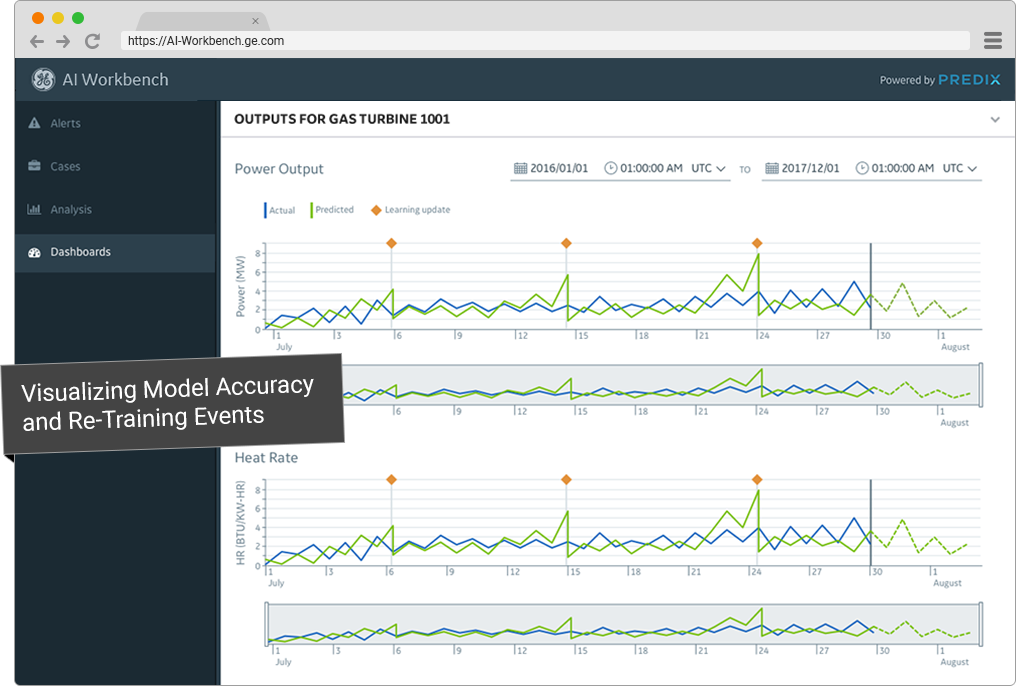